Detecting the efficient integer assignments of multiobjective mixed integer nonlinear programming problems
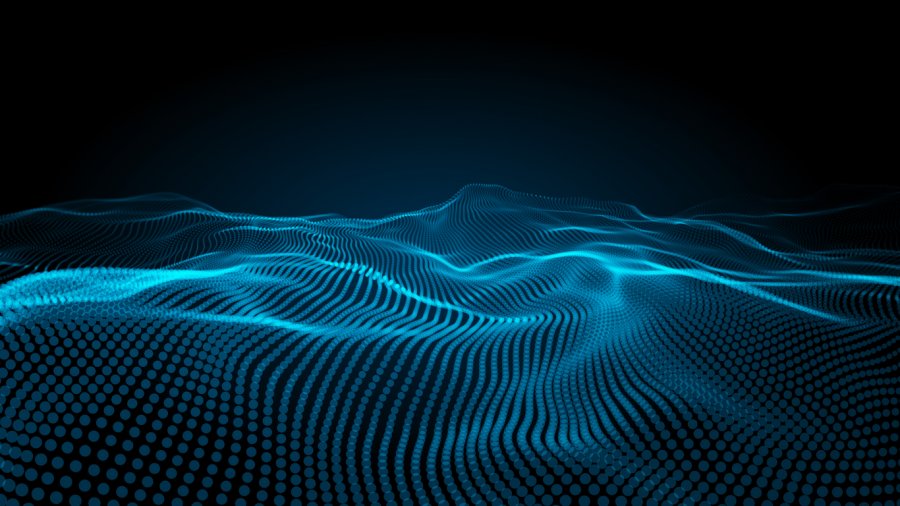
Speaker: Prof. Marianna De Santis
University of Florence
DEIB - Beta Room (Bld. 24)
October 14th, 2024 | 11.00 am
Contact: Prof. Pietro Luigi Belotti
University of Florence
DEIB - Beta Room (Bld. 24)
October 14th, 2024 | 11.00 am
Contact: Prof. Pietro Luigi Belotti
Abstract
On October 14th, 2024 at 11.00 am the seminar titled "Detecting the efficient integer assignments of multiobjective mixed integer nonlinear programming problems" will take place at DEIB Beta Room (Building 24).
Multiobjective mixed integer nonlinear programming (MOMINLP) represents a powerful tool to model real-world decision problems, as applied problems often reveal multiple, conflicting goals to be optimized and 0-1 or integer variables are needed to model logical relationships or discrete quantities. Solving MOMINLPs means to detect the set of efficient solutions of the problem, i.e. those points such that none of the objective functions can be improved in value without degrading some of the other objective values.
An efficient integer assignment for MOMINLPs is a fixing of the integer variables so that there exists an efficient point of the problem with exactly that fixing. Depending on the instance, solution algorithms for MOMINLPs may need to detect a large number of efficient integer assignments, possibly all integer feasible assignments, so that a full enumeration cannot be avoided. This shows a big difference with respect to the single objective case and a big challenge in terms of algorithms development. In this talk, we present a branch-and-bound method for multiobjective mixed-integer convex quadratic programs that computes a superset of efficient integer assignments using outer approximations of the upper image set of continuous relaxations. These outer approximations are obtained addressing the dual formulations of specific subproblems where the values of certain integer variables are fixed. The devised pruning conditions and a tailored preprocessing phase allow a fast enumeration of the nodes. Despite the fact that we do not require any boundedness of the feasible set, we are able to prove that the method stops after having explored a finite number of nodes. Numerical experiments on instances with two, three, and four objectives are presented.
Marianna De Santis is associate professor at University of Florence.
She prior was associate professor at Sapienza University of Rome, where she also got her PhD in Operations Research in 2012 under the supervision of Prof. Stefano Lucidi.
Before coming back to Italy in 2017, she spent some years as post-doc having the chance to work at the Technical University of Dortmund and at the Alpen Adria University of Klagenfurt. Her main research interests are on large-scale nonlinear, mixed integer and multi-objective optimization.
Multiobjective mixed integer nonlinear programming (MOMINLP) represents a powerful tool to model real-world decision problems, as applied problems often reveal multiple, conflicting goals to be optimized and 0-1 or integer variables are needed to model logical relationships or discrete quantities. Solving MOMINLPs means to detect the set of efficient solutions of the problem, i.e. those points such that none of the objective functions can be improved in value without degrading some of the other objective values.
An efficient integer assignment for MOMINLPs is a fixing of the integer variables so that there exists an efficient point of the problem with exactly that fixing. Depending on the instance, solution algorithms for MOMINLPs may need to detect a large number of efficient integer assignments, possibly all integer feasible assignments, so that a full enumeration cannot be avoided. This shows a big difference with respect to the single objective case and a big challenge in terms of algorithms development. In this talk, we present a branch-and-bound method for multiobjective mixed-integer convex quadratic programs that computes a superset of efficient integer assignments using outer approximations of the upper image set of continuous relaxations. These outer approximations are obtained addressing the dual formulations of specific subproblems where the values of certain integer variables are fixed. The devised pruning conditions and a tailored preprocessing phase allow a fast enumeration of the nodes. Despite the fact that we do not require any boundedness of the feasible set, we are able to prove that the method stops after having explored a finite number of nodes. Numerical experiments on instances with two, three, and four objectives are presented.
Marianna De Santis is associate professor at University of Florence.
She prior was associate professor at Sapienza University of Rome, where she also got her PhD in Operations Research in 2012 under the supervision of Prof. Stefano Lucidi.
Before coming back to Italy in 2017, she spent some years as post-doc having the chance to work at the Technical University of Dortmund and at the Alpen Adria University of Klagenfurt. Her main research interests are on large-scale nonlinear, mixed integer and multi-objective optimization.