Learning-based Methods for Model Predictive Control
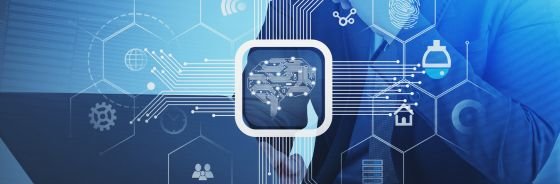
February 9, 2024
09:00 - 10:00 am
Politecnico di Milano - Department of Electronics, Information and Bioengineering
"Emilio Gatti" Conference Room (Building 20)
Speaker: Prof. Alberto Bemporad, IMT School for Advanced Studies Lucca, Italy
Contact: Prof. Lorenzo Fagiano
09:00 - 10:00 am
Politecnico di Milano - Department of Electronics, Information and Bioengineering
"Emilio Gatti" Conference Room (Building 20)
Speaker: Prof. Alberto Bemporad, IMT School for Advanced Studies Lucca, Italy
Contact: Prof. Lorenzo Fagiano
Abstract
Machine learning has gained immense popularity in various fields, including control, due to its ability to extract mathematical models from data. In my talk, I will present different machine learning techniques that can aid in designing and calibrating model predictive control (MPC) laws. I will emphasize the use of offline and online methods for learning piecewise affine and recurrent neural network prediction models. Moreover, I will present global and preference-based optimization techniques that rely on surrogate functions to actively learn the optimal MPC parameters and to identify critical scenarios from closed-loop experiments.
Short Bio
Prof. Alberto Bemporad received his Master's degree cum laude in Electrical Engineering in 1993 and his Ph.D. in Control Engineering in 1997 from the University of Florence, Italy. In 1996/97 he was with the Center for Robotics and Automation, Department of Systems Science & Mathematics, Washington University, St. Louis. In 1997-1999 he held a postdoctoral position at the Automatic Control Laboratory, ETH Zurich, Switzerland, where he collaborated as a Senior Researcher until 2002. In 1999-2009 he was with the Department of Information Engineering of the University of Siena, Italy, becoming an Associate Professor in 2005. In 2010-2011 he was with the Department of Mechanical and Structural Engineering of the University of Trento, Italy. Since 2011 he is Full Professor at the IMT School for Advanced Studies Lucca, Italy, where he served as the Director of the institute in 2012-2015. He spent visiting periods at Stanford University, University of Michigan, and Zhejiang University. In 2011 he co-founded ODYS S.r.l., a company specialized in developing model predictive control systems for industrial production. He has published more than 400 papers in the areas of model predictive control, hybrid systems, optimization, automotive control, and is the co-inventor of 21 patents. He is author or coauthor of various software packages for model predictive control design and implementation, including the Model Predictive Control Toolbox (The Mathworks, Inc.) and the Hybrid Toolbox for MATLAB, and other packages tailored to industrial production. He was an Associate Editor of the IEEE Transactions on Automatic Control during 2001-2004 and Chair of the Technical Committee on Hybrid Systems of the IEEE Control Systems Society in 2002-2010. He received the IFAC High-Impact Paper Award for the 2011-14 triennial, the IEEE CSS Transition to Practice Award in 2019, and the 2021 SAE Environmental Excellence in Transportation Award. He is an IEEE Fellow since 2010.