Handling complexity in large-scale systems via multi-agent decomposition and decentralized optimization
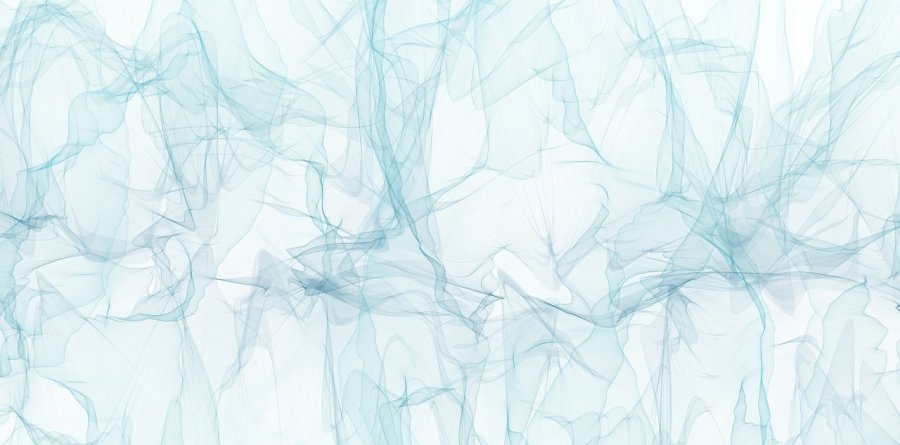
Lucrezia Manieri
PHD Student
DEIB - Conference Room "E. Gatti" (Building 20)
May 17th, 2023
11.30 am
Contacts:
Simone Formentin
Research Line:
Control systems
PHD Student
DEIB - Conference Room "E. Gatti" (Building 20)
May 17th, 2023
11.30 am
Contacts:
Simone Formentin
Research Line:
Control systems
Abstract
On May 17th, 2023 at 11.30 am Lucrezia Manieri, PHD Student in Information Technology, will give a seminar on "Handling complexity in large-scale systems via multi-agent decomposition and decentralized optimization" in DEIB Conference Room.
Motivated by novel applications in the energy domain, we address the optimal operation of large-scale systems comprising both dynamical and logical components. We focus on the case when the problem can be formulated as a mixed integer linear program (MILP) with both discrete and continuous decision variables and propose a resolution scheme that combines multi-agent decomposition and decentralized optimization to defeat combinatorial complexity. The presentation is structured in two parts.
We start by presenting decentralized optimization algorithms for multi-agent constraint coupled MILPs, where each agent solves a reduced order MILP, and a central unit enforces the coupling constraint. The proposed algorithms can find a feasible solution in a finite number of iterations while reducing its sub-optimality level. Then, we address the challenge of disclosing a multi-agent structure hidden in a generic MILP formulation by searching for a suitable permutation of rows and columns of the matrix defining the linear constraints of the program.
Motivated by novel applications in the energy domain, we address the optimal operation of large-scale systems comprising both dynamical and logical components. We focus on the case when the problem can be formulated as a mixed integer linear program (MILP) with both discrete and continuous decision variables and propose a resolution scheme that combines multi-agent decomposition and decentralized optimization to defeat combinatorial complexity. The presentation is structured in two parts.
We start by presenting decentralized optimization algorithms for multi-agent constraint coupled MILPs, where each agent solves a reduced order MILP, and a central unit enforces the coupling constraint. The proposed algorithms can find a feasible solution in a finite number of iterations while reducing its sub-optimality level. Then, we address the challenge of disclosing a multi-agent structure hidden in a generic MILP formulation by searching for a suitable permutation of rows and columns of the matrix defining the linear constraints of the program.