Exact transform-domain variances for collaborative filtering of correlated noise
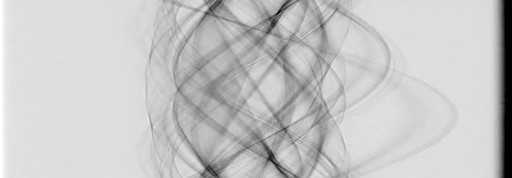
Ymir Mäkinen
Doctoral Researcher
Tampere University - Finland
DEIB - Seminar Room "N. Schiavoni" (Bldg. 20)
November 2nd, 2021
10.00 am
Contacts:
Giacomo Boracchi
Research Line:
System architectures
Doctoral Researcher
Tampere University - Finland
DEIB - Seminar Room "N. Schiavoni" (Bldg. 20)
November 2nd, 2021
10.00 am
Contacts:
Giacomo Boracchi
Research Line:
System architectures
Abstract
On November 2nd, 2021 at 10.00 am Ymir Mäkinen, Tampere University Doctoral Researcher, will hold a seminar on "Exact transform-domain variances for collaborative filtering of correlated noise".
Collaborative filters perform denoising through transform-domain shrinkage of a group of similar blocks extracted from an image. Existing methods for collaborative filtering of stationary correlated noise have all used simple approximations of the transform noise power spectrum adopted from methods which do not employ block grouping. We note the inaccuracies of these approximations and introduce a method for the exact computation and effective approximations of the noise power spectrum. Unlike earlier methods, the calculated noise variances are exact even when noise in one block is correlated with noise in any of the other blocks. We discuss the adoption of the exact noise power spectrum within shrinkage, in similarity testing (block matching), and in aggregation. Extensive experiments support the proposed method over earlier crude approximations used by image denoising filters such as BM3D, demonstrating dramatic improvement in many challenging conditions. As an application of the exact collaborative variances, we demonstrate the filtering of extremely long-range noise correlation through streak noise in micro-tomography data.
In particular, we show how noise in the projections can be modeled through an additive correlated noise model, and denoised through a multiscale framework embedding the improved collaborative filter. We further demonstrate the usage of the exact transform-domain variances in non-local noise spectrum estimation, enabling a fully blind denoising setup.
Collaborative filters perform denoising through transform-domain shrinkage of a group of similar blocks extracted from an image. Existing methods for collaborative filtering of stationary correlated noise have all used simple approximations of the transform noise power spectrum adopted from methods which do not employ block grouping. We note the inaccuracies of these approximations and introduce a method for the exact computation and effective approximations of the noise power spectrum. Unlike earlier methods, the calculated noise variances are exact even when noise in one block is correlated with noise in any of the other blocks. We discuss the adoption of the exact noise power spectrum within shrinkage, in similarity testing (block matching), and in aggregation. Extensive experiments support the proposed method over earlier crude approximations used by image denoising filters such as BM3D, demonstrating dramatic improvement in many challenging conditions. As an application of the exact collaborative variances, we demonstrate the filtering of extremely long-range noise correlation through streak noise in micro-tomography data.
In particular, we show how noise in the projections can be modeled through an additive correlated noise model, and denoised through a multiscale framework embedding the improved collaborative filter. We further demonstrate the usage of the exact transform-domain variances in non-local noise spectrum estimation, enabling a fully blind denoising setup.
Short Bio
Ymir Mäkinen received the B.Sc. and M.Sc. (Tech) degrees from the Tampere University of Technology, Tampere, Finland, in 2017.
He is currently a doctoral researcher with Tampere University, specializing in image restoration.
Register here to participate in presence.
Please, note: COVID-19 Green Pass is needed to attend the event in presence.