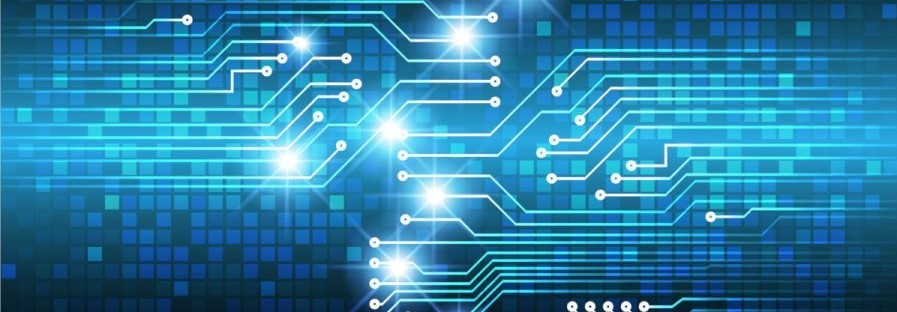
DEIB PhD student
DEIB- Alpha Room (Bldg. 24, Ground Floor)
Event in person and on-line via Webex
October 27th, 2021
5.00 pm
Contacts
Federica Filippini
Research Line
Advanced software architectures and methodologies
In recent years, the great potential of Reinforcement Learning (RL) algorithms has been highlighted by the promising results obtained on simulations and games [1, 2, 3]. The aim of such methods is to define effective policies that describe how an agent should act within a given environment, optimizing a user-defined reward function. The policy design process is based on iteratively collecting experience through the interaction with the environment, where a different reward corresponds to each possible action (or sequence of actions). In many settings, such an iterative process is performed online; however, this is impractical in some real-life scenarios, where data collection is too expensive or dangerous.
The offline RL approach has been designed to learn policies from a previously collected dataset, avoiding direct interactions with the environment and therefore reducing the costs and potential risks generated by such direct exploration [4, 5].
Starting from the description of the RL paradigm, I will: 1) provide an introduction to the most widely used RL algorithms, 2) highlight the most important features of the offline RL approach, 3) illustrate some application fields in which offline RL has been successfully implemented.
[1] David Silver, Thomas Hubert, Julian Schrittwieser, Ioannis Antonoglou, Matthew Lai, Arthur Guez, Marc Lanctot et al. "A general reinforcement learning algorithm that masters chess, shogi, and Go through self-play." Science 362, no. 6419 (2018): 1140-1144.
[2] Oriol Vinyals, Igor Babuschkin, Wojciech M. Czarnecki, Michaël Mathieu, Andrew Dudzik, Junyoung Chung, David H. Choi et al. "Grandmaster level in StarCraft II using multi-agent reinforcement learning." Nature 575, no. 7782 (2019): 350-354.
[3] Christopher Berner, Greg Brockman, Brooke Chan, Vicki Cheung, Przemysław Dębiak, Christy Dennison, David Farhi et al. "Dota 2 with large scale deep reinforcement learning." arXiv preprint arXiv:1912.06680 (2019).
[4] Sergey Levine, Aviral Kumar, George Tucker, Justin Fu, “Offline Reinforcement Learning: Tutorial, Review, and Perspectives on Open Problems.”, arXiv:2005.01643 (2020).
[5] Çağlar Gülçehre, “Deep Reinforcement Learning in the Real World: Offline RL.”, 4th International School on Deep Learning (DeepLearn 2021 Summer), Las Palmas de Gran Canaria, Spain - July 26-30, 2021
The event can be attended:
- in person (only for the Politecnico di Milano personnel)
- remotely/on-line (open to everyone interested) via Webex