High-dimensional MCMC algorithms for Bayesian models
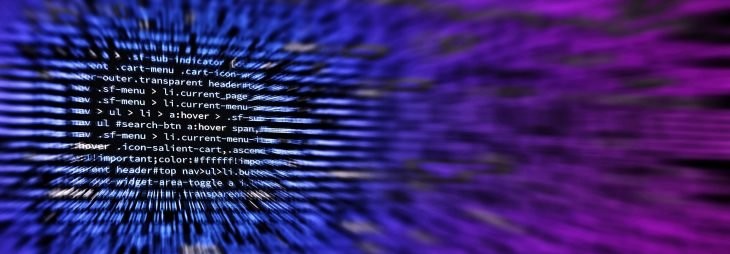
Alessandra Guglielmi, Mario Beraha
DMAT - Politecnico di Milano
Politecnico di Milano - online event organized via Microsoft Teams
September 21st, 2021
4.00 pm
Contacts:
Elisabetta Di Nitto
Research Line:
Advanced software architectures and methodologies
DMAT - Politecnico di Milano
Politecnico di Milano - online event organized via Microsoft Teams
September 21st, 2021
4.00 pm
Contacts:
Elisabetta Di Nitto
Research Line:
Advanced software architectures and methodologies
Abstract
On September 21st, 2021 at 4.00 pm, within the context of the DataCloud activities, Proff. Alessandra Guglielmi and Mario Beraha of DMAT Department - Politecnico di Milano, will hold the online seminar titled "High-dimensional MCMC algorithms for Bayesian models".
In this talk we will introduce the Bayesian approach to inferential statistics and sketch Markov chain Monte Carlo (MCMC) algorithms, that are a class of simulation methods typically used to approximate integrals with respect to probability measures involved in posterior inferences. In general, Bayesian models consider a very large number of parameters, and hence MCMC algorithms with a highly dimensional parameter space need to be designed, with extremely large associated computational cost. As an illlustration we will consider some applications where the goal is (model-based) clustering the data. We use Bayesian mixture models and we will see that, in case of high-dimensional data, the corresponding MCMC algorithms are computationally very demanding.
The event will be held online by Microsoft Teams
In this talk we will introduce the Bayesian approach to inferential statistics and sketch Markov chain Monte Carlo (MCMC) algorithms, that are a class of simulation methods typically used to approximate integrals with respect to probability measures involved in posterior inferences. In general, Bayesian models consider a very large number of parameters, and hence MCMC algorithms with a highly dimensional parameter space need to be designed, with extremely large associated computational cost. As an illlustration we will consider some applications where the goal is (model-based) clustering the data. We use Bayesian mixture models and we will see that, in case of high-dimensional data, the corresponding MCMC algorithms are computationally very demanding.
The event will be held online by Microsoft Teams