An introduction to Reinforcement Learning in Real World
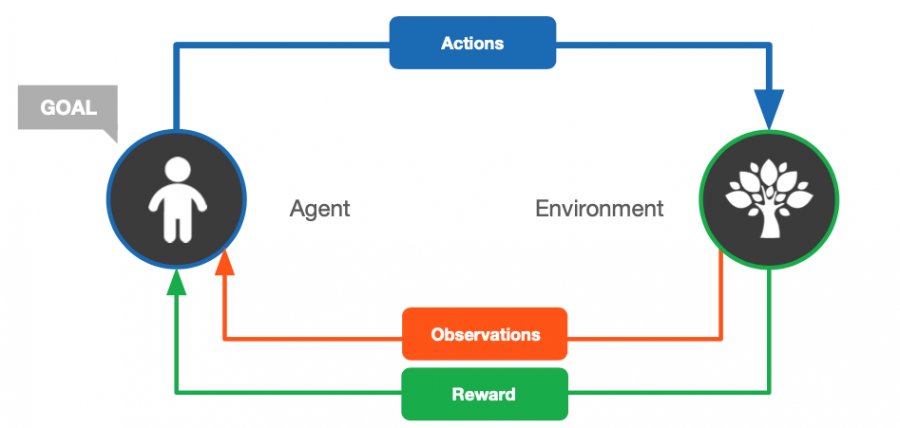
Marco Mussi
PhD student, DEIB
Politecnico di Milano - online event organized via Microsoft Teams
September 3rd, 2021
3.30 pm
Contacts:
Marco Mussi
Research Line:
Artificial intelligence and robotics
PhD student, DEIB
Politecnico di Milano - online event organized via Microsoft Teams
September 3rd, 2021
3.30 pm
Contacts:
Marco Mussi
Research Line:
Artificial intelligence and robotics
Abstract
On September 3rd, 2021 at 3.30 pm Marco Mussi, PhD student of DEIB, will hold an online seminar titled "An introduction to Reinforcement Learning in Real World".
Nowadays, among all the Machine Learning subfields, Reinforcement Learning (RL) is the most investigated by the computer science community. Indeed, these kinds of algorithms are capable of learning optimal policies to perform a specific task, thus they are of interest for many applicative problems. Commonly, RL algorithms need to explore the all the states characterizing the task, gather experience, and use such experience to learn a suitable set of actions able to complete the task in an optimal way.
Even if the research in this field has a long history, most of the developed techniques required, to work properly, a set of assumptions that are hardly met in real-world scenarios. In recent years, to meet the desire and need of the industrial world, a new kind of RL algorithms that can better fit real-world cases have been developed, i.e., the ones based on neural networks and Offline RL methods.
In this seminar, we will cover a brief summary of the classical RL field, and, after that, we will describe some of the most relevant works in the field of Offline RL algorithms developed in the last few years.
The event will be held online via Microsoft Teams.
Nowadays, among all the Machine Learning subfields, Reinforcement Learning (RL) is the most investigated by the computer science community. Indeed, these kinds of algorithms are capable of learning optimal policies to perform a specific task, thus they are of interest for many applicative problems. Commonly, RL algorithms need to explore the all the states characterizing the task, gather experience, and use such experience to learn a suitable set of actions able to complete the task in an optimal way.
Even if the research in this field has a long history, most of the developed techniques required, to work properly, a set of assumptions that are hardly met in real-world scenarios. In recent years, to meet the desire and need of the industrial world, a new kind of RL algorithms that can better fit real-world cases have been developed, i.e., the ones based on neural networks and Offline RL methods.
In this seminar, we will cover a brief summary of the classical RL field, and, after that, we will describe some of the most relevant works in the field of Offline RL algorithms developed in the last few years.
The event will be held online via Microsoft Teams.