Multi-Model Fitting via clustering in preference space
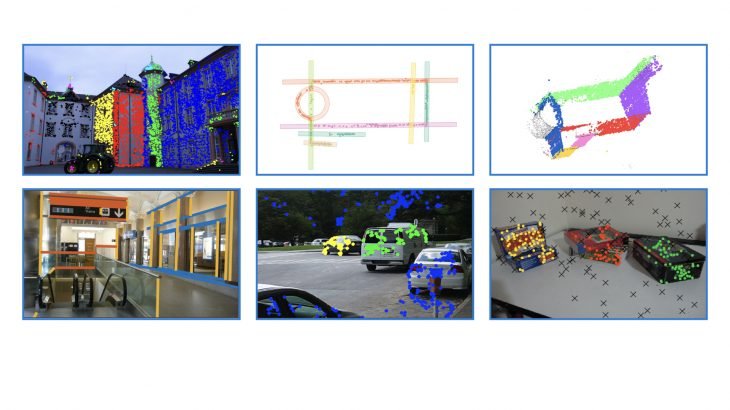
Luca Magri
Politecnico di Milano, DEIB
DEIB - Seminar Room "N. Schiavoni" (building 20)
December 9th, 2019
4.00 pm
Contacts:
Giacomo Boracchi
Research Line:
System architectures
Politecnico di Milano, DEIB
DEIB - Seminar Room "N. Schiavoni" (building 20)
December 9th, 2019
4.00 pm
Contacts:
Giacomo Boracchi
Research Line:
System architectures
Abstract
Many tasks in empirical sciences can be formulated in terms of robust estimation of multiple parametric models (or structures) that fit data corrupted by noise and outliers. Typical examples can be found in 3D reconstruction, where multi-model fitting is employed either to estimate multiple rigid moving objects, or to produce intermediate geometric interpretations of reconstructed 3D point clouds. Other scenarios in which the estimation of multiple geometric models plays a primary role include face clustering, body-pose estimation and motion segmentation, just to name a few. In all these cases, this turns to be a thorny problem since it is necessary to overcome a “chicken-&-egg dilemma”: to estimate models one needs to first partition the data, and to partition the data it is necessary to know which model points belong to. According to which horn of this dilemma is addressed first, two main approaches can be singled out, namely consensus and preference analysis. Consensus-based algorithms put the emphasis on the estimation part and the focus is on finding models that describe as many points as possible. In contrast, preference approaches concentrate on the segmentation side, and are aimed at finding a proper partition of the data in meaningful structures. In this talk, we start with reviewing RANSAC, the mainstream single-model fitting algorithm based on consensus maximisation. Then we will see how the change of perspective from consensus to preference allows to derive a conceptual space where the multi model fitting task can be conveniently formulated as a clustering problem. Specifically, I will present T-Linkage [Magri and Fusiello. “T-linkage: A continuous relaxation of J-linkage for multi-model fitting.” CVPR14, Magri and Fusiello. “Fitting Multiple Heterogeneous Models by Multi-Class Cascaded T-Linkage” CVPR19], a hierarchical clustering method for preference analysis, that have been successfully employed in several Computer Vision applications. Examples which I will illustrate includes Computer Vision tasks based on the estimation of underlying geometric models, e.g. plane detection, vanishing points estimation and multi-view motion segmentation.
Short Bio
Luca Magri received the Laurea (M.S.) degree in Mathematics and the Dottorato di Ricerca (Ph.D) degree in Mathematic and Statistic for Computational Sciences from the University of Milano, Italy, in 2011 and 2015, respectively. He has been a postdoc researcher in the University of Verona and Udine where he worked on robust multi model fitting for Computer Vision applications and 3D reconstruction.