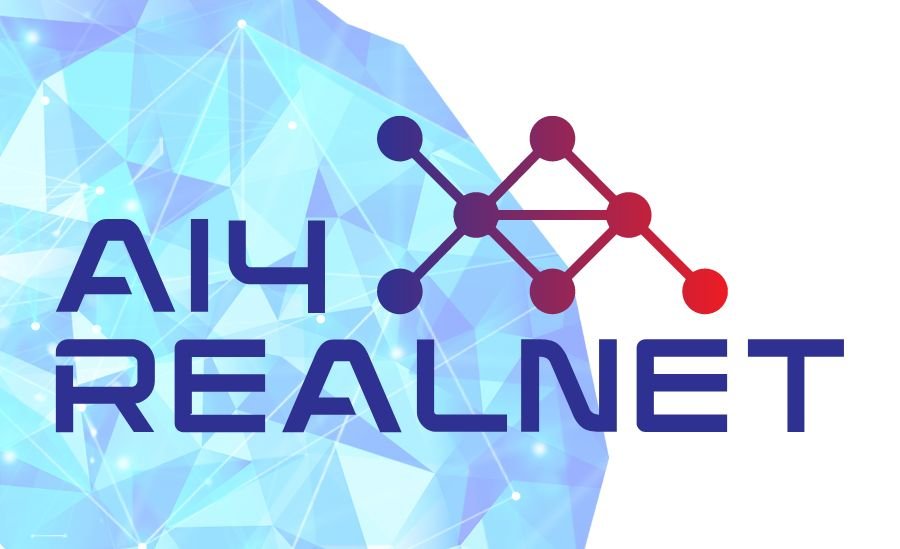
Research Lines:
AI4REALNET - AI for REAL-world NETwork operation main ambition is to successfully create an overarching multidisciplinary approach, combining emerging AI algorithms/concepts, open-source digital environments to test and benchmark AI in industry-driven use cases, socio-technical design of AI-based decision systems and human-machine interaction, to better operate (in predictive and real-time modes) complex network infrastructures.
The research aspects will be further enhanced crosswise along two critical infrastructures whose virtual and physical assets, systems, and networks are considered vital in Europe, and their disruption would have a debilitating effect on society. These infrastructures are energy (electric power grid) and transport (railway and air traffic management), two out of the five priority sectors identified in the European national AI strategies. In the AI4REALNET vision, high levels of human control and AI-based automation coexist with the “optimal” balance and are divided into a) full human control (AI-assisted), b) co-learning between AI and human, and c) trustworthy (human certified) full AI-based control.
It will develop research towards boosted teaming between AI and humans through well-redesigned (with AI) mission-critical tasks in industry, where this cooperation empowers humans, improves human performance, delivers higher levels of reliability and safety of the critical infrastructures by avoiding excessive automation or human control.
The fundamental elements are: a) AI algorithms mainly composed of reinforcement learning and supervised learning, unifying the benefits of existing heuristics, physical modelling of these complex systems and learning methods, as well as a set of complementary techniques to enhance transparency, safety, explainability, and human acceptance of these algorithms; b) human-in-the-loop decision making that promotes co-learning between AI and humans, considering timely challenges like integration of model uncertainty, risk, human cognitive load, and trust; c) safe autonomous AI systems relying on human supervision, embedded with human domain knowledge, safety rules and limits defined by physical equations in the AI learning phase.