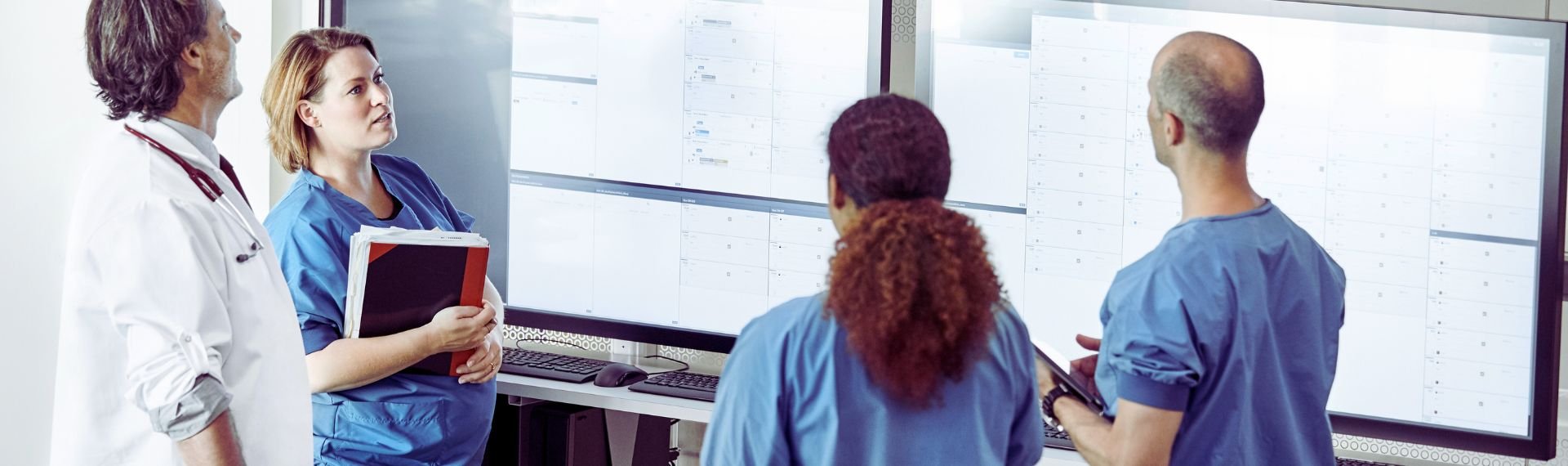
Validating "Personas" — fictional profiles used to represent different user types — is often a complex and expensive process, especially in fields like healthcare. But a new study, co-authored by Emanuele Tauro and Enrico Caiani from the Department of Electronics, Information and Bioengineering – Politecnico di Milano, has introduced a smarter, more cost-effective solution.
The team developed a new approach that uses artificial intelligence, specifically a technique called self-supervised machine learning. What makes it unique is that it relies only on the data already collected when Personas are created, eliminating the need for costly extra validation steps.
Here's how it works in simple terms: the researchers used part of the data (80%) to create Persona and cluster users based on similarity. They then ran multiple AI models that learned their characteristics. Subsequently, models were tested on the remaining 20%, using a voting system, to see if they could predict the correct Persona for each individual. They then measured how well the system worked using standard metrics like accuracy and precision.
The method was tested on two very different healthcare datasets based on questionnaire responses. The first included over 1,000 people, while the second involved 176. Despite differences in size and balance between groups, the method consistently showed high performance — accurately identifying Persona groups more than 88% of the time.
Most importantly, this approach doesn’t just save time and money—it also proves that Personas can meaningfully represent real differences in people’s behaviours and needs. That makes it a powerful tool for healthcare, marketing, and any field where understanding users is key.