NECSTFridayTalk – Guessing As A Service: Large Language Models Are Not Yet Ready For Vulnerability Detection
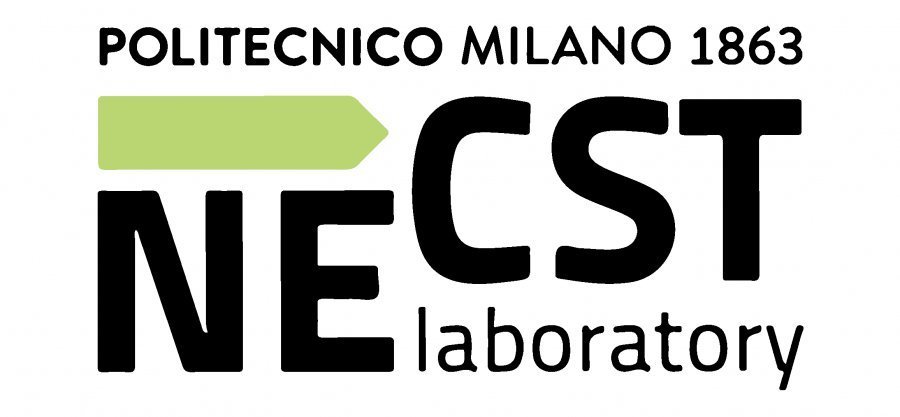
Speaker: Francesco Panebianco
PHD Student in Information Technology
DEIB - NECSTLab Meeting Room (Bld. 20)
Online by Zoom
May 16th, 2025 | 11.30 am
Contact: Prof. Marco Santambrogio
PHD Student in Information Technology
DEIB - NECSTLab Meeting Room (Bld. 20)
Online by Zoom
May 16th, 2025 | 11.30 am
Contact: Prof. Marco Santambrogio
Sommario
On Friday, May 16th, 2025 at 11.30 am a new appointment of #NECSTFridayTalk series titled "Guessing As A Service: Large Language Models Are Not Yet Ready For Vulnerability Detection" will take place at DEIB NECSTLab Meeting Room (Building 20) and on line by Zoom.
During this talk, we will have, as speaker, Francesco Panebianco, PhD student at Dipartimento di Elettronica, Informazione e Bioingegneria.
The growing number of reported software vulnerabilities underscores the need for efficient detection methods, especially for resource-limited organizations. While traditional techniques like fuzzing and symbolic execution are effective, they require significant manual effort. Recent advances in Large Language Models (LLMs) show promise for zero-shot learning, leveraging pre-training on diverse datasets to detect vulnerabilities without fine-tuning. This study evaluates quantized models, code-specialized models, and fine-tuned approaches. Zero-shot models perform poorly, and fine-tuned alternatives are undermined by overfitting. These findings emphasize the limitations of current AI solutions and the necessity for approaches tailored to the specific problem.
During this talk, we will have, as speaker, Francesco Panebianco, PhD student at Dipartimento di Elettronica, Informazione e Bioingegneria.
The growing number of reported software vulnerabilities underscores the need for efficient detection methods, especially for resource-limited organizations. While traditional techniques like fuzzing and symbolic execution are effective, they require significant manual effort. Recent advances in Large Language Models (LLMs) show promise for zero-shot learning, leveraging pre-training on diverse datasets to detect vulnerabilities without fine-tuning. This study evaluates quantized models, code-specialized models, and fine-tuned approaches. Zero-shot models perform poorly, and fine-tuned alternatives are undermined by overfitting. These findings emphasize the limitations of current AI solutions and the necessity for approaches tailored to the specific problem.
The NECSTLab is a DEIB laboratory, with different research lines on advanced topics in computing systems: from architectural characteristics, to hardware-software codesign methodologies, to security and dependability issues of complex system architectures.
Every week, the “NECSTFridayTalk” invites researchers, professionals or entrepreneurs to share their work experiences and projects they are implementing in the “Computing Systems”.