NECSTFridayTalk - AI Hardware Accelerators: Integrating In-Memory Computing into RISC-V for Scalable Deep Learning
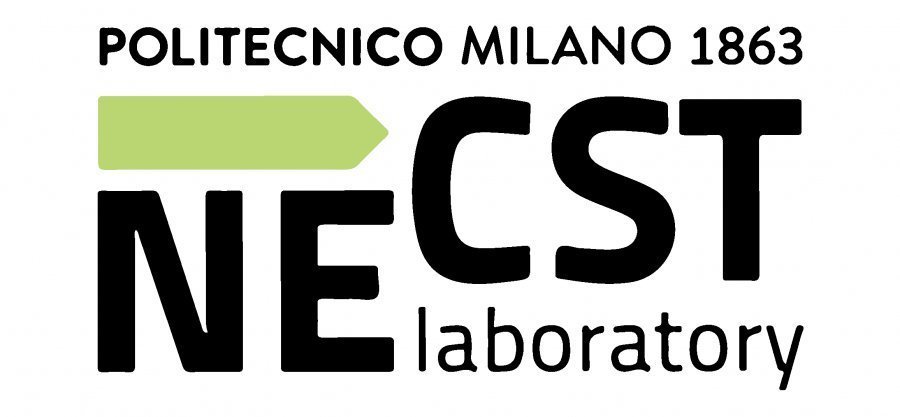
May 23, 2025 | 11:30 a.m.
DEIB - NECSTLab Meeting Room (Bld. 20)
Online on Zoom
Speaker: Tommaso Spagnolo (Politecnico di Milano)
Contacts: Prof. Marco Santambrogio | marco.santambrogio@polimi.it
DEIB - NECSTLab Meeting Room (Bld. 20)
Online on Zoom
Speaker: Tommaso Spagnolo (Politecnico di Milano)
Contacts: Prof. Marco Santambrogio | marco.santambrogio@polimi.it
Sommario
On Friday, May 23, 2025 at 11:30 a.m. a new appointment of the NECSTFridayTalk series, titled "AI Hardware Accelerators: Integrating In-Memory Computing into RISC-V for Scalable Deep Learning" will take place in the NECSTLab Meeting Room (Building 20) and online on Zoom.
Tommaso Spagnolo, PhD student at the Department of Electronics, Information and Bioengineering, will be the speaker.
Tommaso Spagnolo, PhD student at the Department of Electronics, Information and Bioengineering, will be the speaker.
As the demand for efficient AI processing continues to grow, conventional hardware architectures struggle to meet the energy and latency constraints of modern deep learning applications, especially at the edge. This talk explores the critical role of hardware accelerators in overcoming these limitations, focusing on the synergy between open-source RISC-V architectures and emerging In-Memory Computing (IMC) paradigms.
The speech will begin by outlining the fundamental challenges in AI hardware design and the motivations for integrating IMC units. Then, Tommaso will present his recent research project: Extending the RISC-V Vector Instruction Set Architecture to Integrate Digital In-Memory Computing for Deep Learning Acceleration at the Edge. This work introduces custom instructions and a novel hardware-software co-design approach to seamlessly incorporate IMC into a vector processor, enabling higher throughput and energy efficiency for convolutional neural networks.
In the final part, the vision for the next phase of this research will be discussed: developing a modular and scalable architecture capable of bridging edge and high-performance computing (HPC) domains. This approach aims to unify flexibility, efficiency, and performance across the full spectrum of AI workloads.