Bubble Entropy: a fast and parameter-free entropy estimator
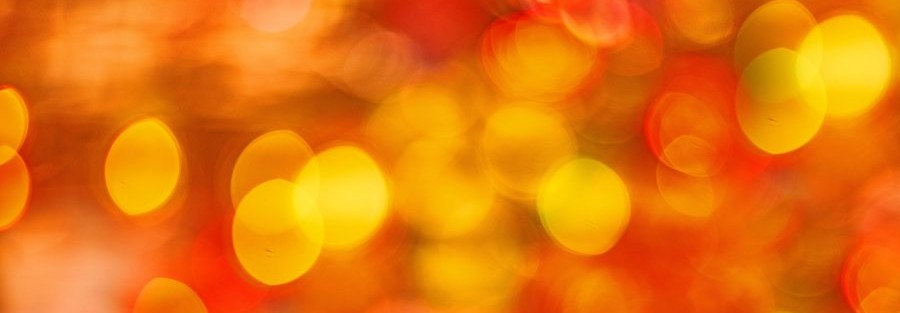
George Manis
Associate Professor, School of Engineering University
Ioannina, Greece
DEIB - Beta Room (Building 24)
Via Golgi 40, Milano
Contacts:
Sergio Cerutti
Associate Professor, School of Engineering University
Ioannina, Greece
DEIB - Beta Room (Building 24)
Via Golgi 40, Milano
December 13th, 2022
5.00 pm
Contacts:
Sergio Cerutti
Sommario
On December 13th, 2022 at 5.00 pm George Manis, Associate Professor at Department of Computer Science and Engineering, School of Engineering University of Ioannina (Greece), will give a seminar on "Bubble Entropy: a fast and parameter-free entropy estimator" in DEIB Beta Room.
Entropy analysis is a powerful tool in various areas of signal interpretation. In particular, significant applications of entropy can be found in many biomedical engineering (BME) fields, including cardiovascular and neurosensorial signal analysis, cancer research, sleep disorders and gait analysis. Bubble Entropy is a recently proposed definition of entropy. Having certain advantages over more diffused definitions, Bubble Entropy finds its place in the research community map. It belongs to the family of entropy estimators which embed the signal into a m-dimensional space and estimate entropy on the trajectory in this space. Two are the main drawbacks for which those methods are criticized: the high computational cost and the dependence on parameters. Bubble Entropy can be an answer to both, since computation can be done in linear time and the dependence on parameters can be considered as minimal or even zero. The most diffused entropy definitions mainly rely on two parameters: the size of the embedding space m and the threshold distance r, which refers to the distance between two points in the m-dimensional space. Bubble Entropy totally eliminates the necessity to define a threshold distance, while m is fundamental in the concept of embedding signals in higher dimensional spaces, and therefore it cannot be eliminated.
On the other hand, we show that the selection of m is less important here than in any other method of entropy estimation and why Bubble Entropy can discriminate between groups of subjects, without having to decide about the size of the embedding space. In this seminar, the presented experimental results are relative to congestive heart failure (HF) subjects and controls and it is shown that Bubble Entropy does not lack discriminating power, compared to the most diffused, well established, entropy estimators.
Entropy analysis is a powerful tool in various areas of signal interpretation. In particular, significant applications of entropy can be found in many biomedical engineering (BME) fields, including cardiovascular and neurosensorial signal analysis, cancer research, sleep disorders and gait analysis. Bubble Entropy is a recently proposed definition of entropy. Having certain advantages over more diffused definitions, Bubble Entropy finds its place in the research community map. It belongs to the family of entropy estimators which embed the signal into a m-dimensional space and estimate entropy on the trajectory in this space. Two are the main drawbacks for which those methods are criticized: the high computational cost and the dependence on parameters. Bubble Entropy can be an answer to both, since computation can be done in linear time and the dependence on parameters can be considered as minimal or even zero. The most diffused entropy definitions mainly rely on two parameters: the size of the embedding space m and the threshold distance r, which refers to the distance between two points in the m-dimensional space. Bubble Entropy totally eliminates the necessity to define a threshold distance, while m is fundamental in the concept of embedding signals in higher dimensional spaces, and therefore it cannot be eliminated.
On the other hand, we show that the selection of m is less important here than in any other method of entropy estimation and why Bubble Entropy can discriminate between groups of subjects, without having to decide about the size of the embedding space. In this seminar, the presented experimental results are relative to congestive heart failure (HF) subjects and controls and it is shown that Bubble Entropy does not lack discriminating power, compared to the most diffused, well established, entropy estimators.
Biografia
George Manis is an Associate Professor of Biomedical Engineering in the Department of Computer Science and Engineering, School of Engineering, University of Ioannina, Greece.
Academic Degrees: He received his first degree (5-years Diploma degree) from the Department of Electrical and Computer Engineering, National Technical University of Athens (1992), his MSc from Queen Mary, University of London (1993) and his PhD from the National Technical University of Athens (1997).
Academic Positions: He served in the Department of Computer Science and Engineering at the University of Ioannina as Adjacent Teaching Stuff (2000), Lecturer (2002), Assistant Professor (2008) and Associate Professor (since 2017). He also teaches in the Hellenic Open University (2000-today).
Research Interests: His research interests include Biomedical Engineering, Biomedical Data Classification and Computing Systems.
Publications: He has published two books and has had the scientific coordination in the editing of two more. He has published more than one hundred papers in scientific Journals and Conferences with various applications to physiological systems.
Scientific Visits: During academic leaves, he has visited the Politecnico di Milano and the Università degli Studi di Milano three times, in 2009, 2015 and 2022.
Academic Degrees: He received his first degree (5-years Diploma degree) from the Department of Electrical and Computer Engineering, National Technical University of Athens (1992), his MSc from Queen Mary, University of London (1993) and his PhD from the National Technical University of Athens (1997).
Academic Positions: He served in the Department of Computer Science and Engineering at the University of Ioannina as Adjacent Teaching Stuff (2000), Lecturer (2002), Assistant Professor (2008) and Associate Professor (since 2017). He also teaches in the Hellenic Open University (2000-today).
Research Interests: His research interests include Biomedical Engineering, Biomedical Data Classification and Computing Systems.
Publications: He has published two books and has had the scientific coordination in the editing of two more. He has published more than one hundred papers in scientific Journals and Conferences with various applications to physiological systems.
Scientific Visits: During academic leaves, he has visited the Politecnico di Milano and the Università degli Studi di Milano three times, in 2009, 2015 and 2022.