Tackling difficult engineering design problems: a Set Membership approach
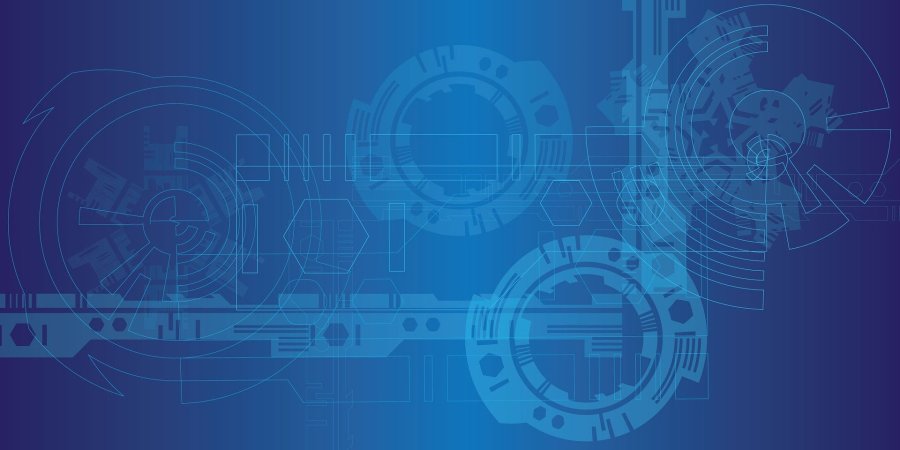
Lorenzo Jr Sabug
PHD Student in Information Technology
DEIB - Conference Room "E. Gatti" (Building 20)
November 16th, 2022
11.30 am
Contacts:
Lorenzo Jr Sabug
Research Line:
Control systems
PHD Student in Information Technology
DEIB - Conference Room "E. Gatti" (Building 20)
November 16th, 2022
11.30 am
Contacts:
Lorenzo Jr Sabug
Research Line:
Control systems
Sommario
On November 16th, 2022 at 11.30 am Lorenzo Jr Sabug, PHD Student in Information Technology, will give a seminar on "Tackling difficult engineering design problems: a Set Membership approach" in DEIB Conference Room.
In many practical engineering domains, we encounter non-convex design problems in which the objective (and if applicable, also constraints) is not explicitly available through mathematical expressions. It may be because of the difficulty in modelling the system at hand, associated with the mixed nature of the physical quantities involved. In some other cases, this is made more complex by the non-trivial interactions of the mechanisms with each other and the environment. Hence, evaluating the objective entails performing time-consuming experiments or simulations, for which only the objective values are available, i.e. there is no gradient information available. To solve these problems, data-driven optimization methods are usually used, strategically choosing the next sampling points from the currently available ones. In this talk, a new data-driven optimization approach using a Set Membership framework will be discussed. It automatically trades off between exploiting information about the current best point found so far, and exploring the search space for better points. The algorithm flow will be discussed, with a short overview of its theoretical and computational properties. We demonstrate the effectiveness of the optimization approach to an illustrative problem of MPC tuning for a servomotor with uncertain parameters.
In many practical engineering domains, we encounter non-convex design problems in which the objective (and if applicable, also constraints) is not explicitly available through mathematical expressions. It may be because of the difficulty in modelling the system at hand, associated with the mixed nature of the physical quantities involved. In some other cases, this is made more complex by the non-trivial interactions of the mechanisms with each other and the environment. Hence, evaluating the objective entails performing time-consuming experiments or simulations, for which only the objective values are available, i.e. there is no gradient information available. To solve these problems, data-driven optimization methods are usually used, strategically choosing the next sampling points from the currently available ones. In this talk, a new data-driven optimization approach using a Set Membership framework will be discussed. It automatically trades off between exploiting information about the current best point found so far, and exploring the search space for better points. The algorithm flow will be discussed, with a short overview of its theoretical and computational properties. We demonstrate the effectiveness of the optimization approach to an illustrative problem of MPC tuning for a servomotor with uncertain parameters.