Multi-trajectory Model Predictive Control for constrained navigation of autonomous vehicles
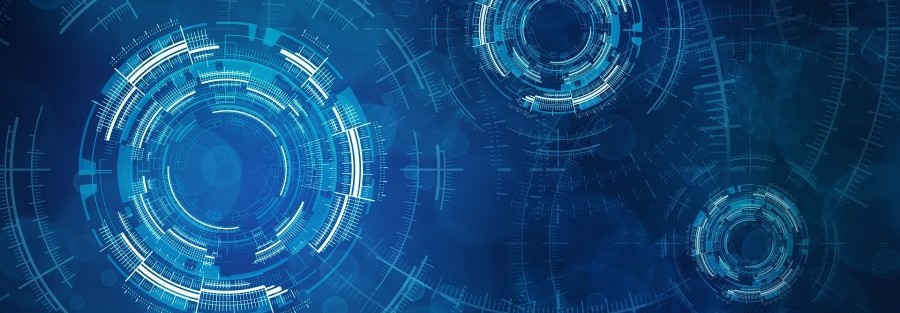
Danilo Saccani
PHD Student in Information Technology
DEIB - Conference Room "E. Gatti" (Building 20)
November 16th, 2022
11.30 am
Contacts:
Danilo Saccani
Research Line:
Control systems
PHD Student in Information Technology
DEIB - Conference Room "E. Gatti" (Building 20)
November 16th, 2022
11.30 am
Contacts:
Danilo Saccani
Research Line:
Control systems
Sommario
On November 16th, 2022 at 11.30 am Danilo Saccani, PHD Student in Information Technology, will give a seminar on "Multi-trajectory Model Predictive Control for constrained navigation of autonomous vehicles" in DEIB Conference Room.
The autonomous navigation of vehicles in cluttered unknown environments is still an open challenge, particularly when safety requirements must be met. Among the different approaches for dynamic path planning, discrete optimization approaches, such as Model Predictive Control (MPC) schemes, have received broad attention thanks to their ability to manage state and input constraints while minimizing a user-defined cost function.
The design of motion planning algorithms for safety-critical applications must trade-off between safety, here considered in the form of constraint satisfaction and persistent obstacle avoidance, and exploitation, i.e. to reach the desired target in a short time.
To deal with this balancing issue, in this seminar, we propose a novel MPC approach that we find particularly suitable for time-varying constraints, named multi-trajectory MPC (mt-MPC). To trade-off safety and exploitation in an intuitive way, mt-MPC considers different future state trajectories in the same Finite Horizon Optimal Control Problem (FHOCP), enabling a partial decoupling between constraint satisfaction (safety) and cost function minimization (exploitation). After a presentation of the approach, we will see its application for the autonomous navigation of a drone in an unknown environment.
The autonomous navigation of vehicles in cluttered unknown environments is still an open challenge, particularly when safety requirements must be met. Among the different approaches for dynamic path planning, discrete optimization approaches, such as Model Predictive Control (MPC) schemes, have received broad attention thanks to their ability to manage state and input constraints while minimizing a user-defined cost function.
The design of motion planning algorithms for safety-critical applications must trade-off between safety, here considered in the form of constraint satisfaction and persistent obstacle avoidance, and exploitation, i.e. to reach the desired target in a short time.
To deal with this balancing issue, in this seminar, we propose a novel MPC approach that we find particularly suitable for time-varying constraints, named multi-trajectory MPC (mt-MPC). To trade-off safety and exploitation in an intuitive way, mt-MPC considers different future state trajectories in the same Finite Horizon Optimal Control Problem (FHOCP), enabling a partial decoupling between constraint satisfaction (safety) and cost function minimization (exploitation). After a presentation of the approach, we will see its application for the autonomous navigation of a drone in an unknown environment.