Learning 𝛿ISS RNN models for Model Predictive Control: a small step towards theory-friendly RNNs for control design
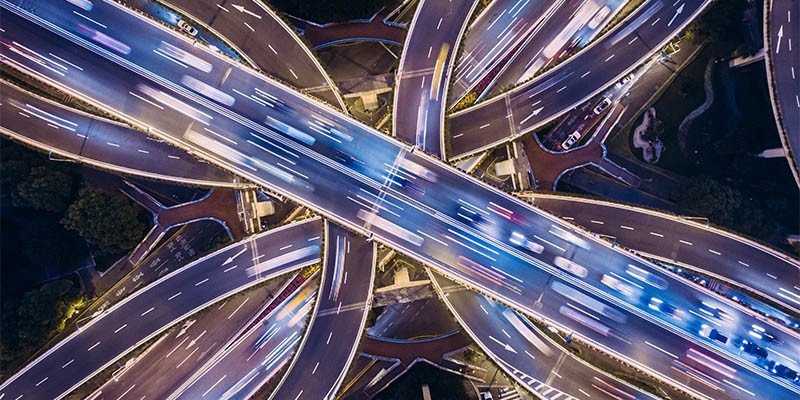
Fabio Bonassi
DEIB PHD Student
DEIB - Conference Room (Building 20)
September 16th, 2022
11.30 am
Contacts:
Fabio Bonassi
Research Line:
Control systems
DEIB PHD Student
DEIB - Conference Room (Building 20)
September 16th, 2022
11.30 am
Contacts:
Fabio Bonassi
Research Line:
Control systems
Sommario
On September 16th, 2022 at 11.30 am Fabio Bonassi, PHD Student in Information Technology, will hold a seminar on "Learning 𝛿ISS RNN models for Model Predictive Control: a small step towards theory-friendly RNNs for control design" in DEIB Conference Room.
In this presentation we discuss the synthesis of predictive control laws based on Recurrent Neural Networks (RNNs) models that enjoy the Incremental Input-to-State Stability (δISS) property. To this end, we first show how to train RNNs that are provably δISS to identify stable unknown dynamical systems. Then, such stability property is shown to guarantee the closed-loop stability of suitably synthesize predictive control laws based on these RNN models.
In this presentation we discuss the synthesis of predictive control laws based on Recurrent Neural Networks (RNNs) models that enjoy the Incremental Input-to-State Stability (δISS) property. To this end, we first show how to train RNNs that are provably δISS to identify stable unknown dynamical systems. Then, such stability property is shown to guarantee the closed-loop stability of suitably synthesize predictive control laws based on these RNN models.