Self-supervised learning in seismic data processing
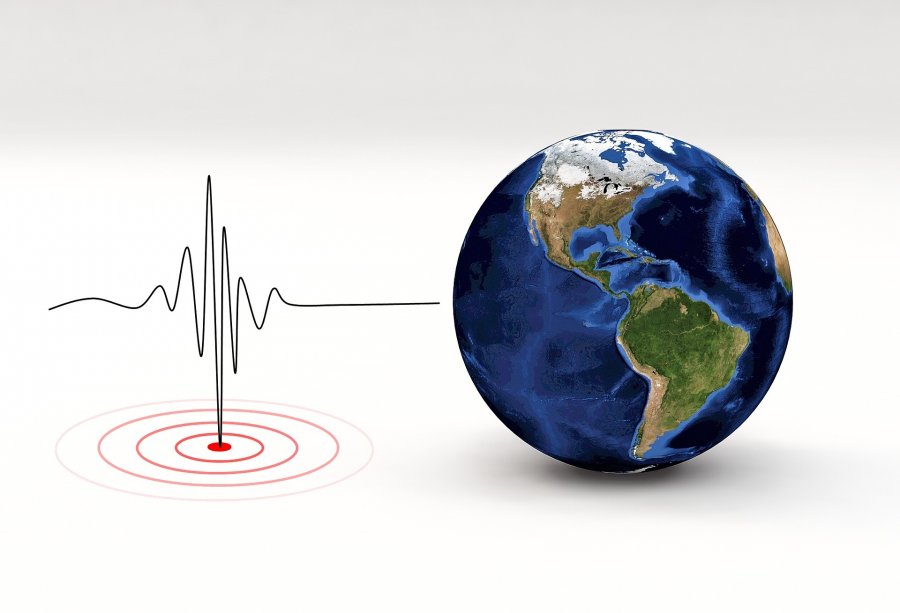
Matteo Ravasi
KAUST
DEIB - Alpha Room (Building 24)
May 31st, 2022
9.00 am
Contacts:
Marco Marcon
Research Line:
Signal processing for multimedia and telecommunications
KAUST
DEIB - Alpha Room (Building 24)
May 31st, 2022
9.00 am
Contacts:
Marco Marcon
Research Line:
Signal processing for multimedia and telecommunications
Sommario
On May 31st, 2022 at 9.00 am, Prof. Matteo Ravasi, KAUST - King Abdullah University of Science and Technology, School of Earth Science, Arabia Saudita, will hold a seminar on
"Self-supervised learning in seismic data processing" in DEIB Alpha Room.
Deep learning has taken the field of geophysics by storm. However, after the initial excitement accompanied by a variety of successful applications in interpretative tasks, the subsequent wave of solutions for seismic processing and imaging has so far not delivered as intended. The main reasons behind this initial unsuccess are: i) the fact that processing and imaging are likely to be framed as regression (or domain translation) tasks, where signal preservation is a must; ii) the lack of trustworthy ‘noisy-clean’ training pairs, and; iii) the inability to explicitly take into account the underlying physical process associated to a given processing task. A paradigm shift is therefore required where reliance on training data is relaxed and the physical process is included as part of the learning algorithm. In this talk,
I will discuss a number of applications that my group is currently developing combining so-called self-supervised learning with classical inverse problem theory to solve seismic processing tasks ranging from denoising to wavefield separation and interpolation to simultaneous source deblending.
"Self-supervised learning in seismic data processing" in DEIB Alpha Room.
Deep learning has taken the field of geophysics by storm. However, after the initial excitement accompanied by a variety of successful applications in interpretative tasks, the subsequent wave of solutions for seismic processing and imaging has so far not delivered as intended. The main reasons behind this initial unsuccess are: i) the fact that processing and imaging are likely to be framed as regression (or domain translation) tasks, where signal preservation is a must; ii) the lack of trustworthy ‘noisy-clean’ training pairs, and; iii) the inability to explicitly take into account the underlying physical process associated to a given processing task. A paradigm shift is therefore required where reliance on training data is relaxed and the physical process is included as part of the learning algorithm. In this talk,
I will discuss a number of applications that my group is currently developing combining so-called self-supervised learning with classical inverse problem theory to solve seismic processing tasks ranging from denoising to wavefield separation and interpolation to simultaneous source deblending.
Biografia
Matteo is an Assistant Professor at KAUST in the School of Earth Science and Engineering. Prior to that, Matteo held a variety of roles in Equinor both within research and operations. He holds a Phd in Geophysics from the University of Edinburgh and an MSc and BSc in Telecommunication Engineering from Politecnico di Milano.
Matteo has made several contributions in the areas of seismic processing and imaging by developing novel methods to improve the quality and resolution of subsurface imaging products. For his work, Matteo is the recipient of the SEG Karcher Award, RAS Keith Runcorn Prize, and Gustavo Sclocchi Theses Award. He is also the inventor of 2 international patents and author of 18 peer reviewed papers. Matteo is heavily involved in the development of the open-source Python framework for large-scale inverse problems PyLops.