Learning-based compression of immersive video
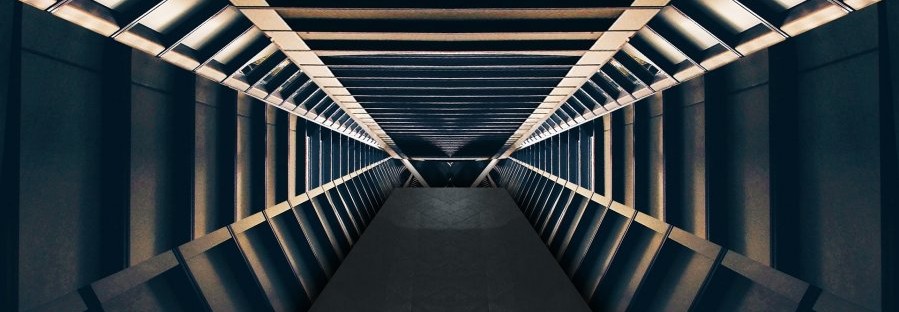
Giuseppe Valenzise
CNRS
DEIB - Seminar Room "N. Schiavoni" (Building 20)
May 30th, 2022
4.00 pm
Contacts:
Marco Marcon
Research Line:
Signal processing for multimedia and telecommunications
CNRS
DEIB - Seminar Room "N. Schiavoni" (Building 20)
May 30th, 2022
4.00 pm
Contacts:
Marco Marcon
Research Line:
Signal processing for multimedia and telecommunications
Sommario
On May 30th, 2022 at 4.00 pm, Prof. Giuseppe Valenzise, CNRS - CentraleSupelec, Université Paris-Saclay, will give a seminar on "Learning-based compression of immersive video" in DEIB Seminar Room.
Immersive video aims at producing realistic and interactive visual experiences by providing a higher degree of realism and interaction for users. Immersive video representations such as omnidirectional (360-degree) video, light fields (LF) and 3D point clouds (PC), require significant amounts of bandwidth and storage space. As a result, their use in practical use cases depends on the availability of efficient coding tools, which has motivated standardization efforts by both MPEG and JPEG. In this talk, I will present recent work on lossy and lossless compression of light fields and 3D point clouds using deep learning. After an introduction to these data representations, I will describe a variational autoencoder-based architecture, initially proposed for coding 2D images, and show how it can be extended to lossy LF and PC compression. I will also discuss lossless LF and PC coding by means of auto-regressive generative models. I will conclude with a benchmark of these methods compared with the available standard solutions.
Immersive video aims at producing realistic and interactive visual experiences by providing a higher degree of realism and interaction for users. Immersive video representations such as omnidirectional (360-degree) video, light fields (LF) and 3D point clouds (PC), require significant amounts of bandwidth and storage space. As a result, their use in practical use cases depends on the availability of efficient coding tools, which has motivated standardization efforts by both MPEG and JPEG. In this talk, I will present recent work on lossy and lossless compression of light fields and 3D point clouds using deep learning. After an introduction to these data representations, I will describe a variational autoencoder-based architecture, initially proposed for coding 2D images, and show how it can be extended to lossy LF and PC compression. I will also discuss lossless LF and PC coding by means of auto-regressive generative models. I will conclude with a benchmark of these methods compared with the available standard solutions.
Biografia
Giuseppe Valenzise completed a master degree and a Ph.D. in Information Technology at the Politecnico di Milano, Italy, in 2007 and 2011, respectively. In 2012, he joined the French Centre National de la Recherche Scientifique (CNRS) as a permanent researcher, first at the Laboratoire Traitement et Communication de l'Information (LTCI) Telecom Paristech, and from 2016 at the Laboratoire des Signaux et Systmes (L2S), CentraleSupelec, Université Paris-Saclay. His research interests span different fields of image and video processing, including traditional and learning-based image and video compression, light fields and point cloud coding, image/video quality assessment, high dynamic range imaging and applications of machine learning to image and video analysis. He is co-author of more than 70 research publications and of several award-winning papers. He is the recipient of the EURASIP Early Career Award 2018. Dr. Valenzise serves/has served as Associate Editor for IEEE Transactions on Circuits and Systems for Video Technology, IEEE Transactions on Image Processing, Elsevier Signal Processing: Image communication. He is an elected member of the MMSP and IVMSP technical committees of the IEEE Signal Processing Society for the term 2018-2020, as well as a member of the Technical Area Committee on Visual Information Processing of EURASIP. He is the EURASIP Best PhD Thesis Award chair for 2020.