Reinforcement Learning Approaches for the Orienteering Problem with Stochastic and Dynamic Release Dates
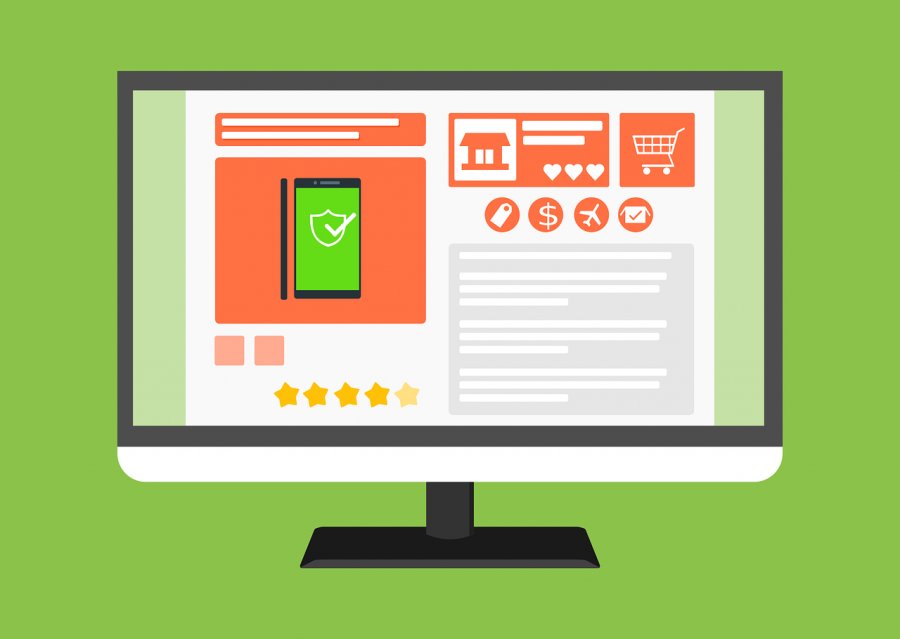
Claudia Archetti
ESSEC Business School
DEIB - PT1 Room (Building 20)
May 27th, 2022
11.00 am
Contacts:
Ola Jabali
Research Line:
Operations research and discrete optimization
ESSEC Business School
DEIB - PT1 Room (Building 20)
May 27th, 2022
11.00 am
Contacts:
Ola Jabali
Research Line:
Operations research and discrete optimization
Sommario
On May 27th, 2022 at 11.00 am, Claudia Archetti (ESSEC Business School), will hold a seminar on "Reinforcement Learning Approaches for the Orienteering Problem with Stochastic and Dynamic Release Dates" in DEIB PT1 Room.
E-commerce markets are booming at remarkable rates. It is reported that the e-commerce revenue saw a 10% increase in Europe in 2020 due to the pandemic. At the same time, the expectations of customers in terms of service quality are also increasing. An important feature of last-mile distribution is that its operations start as soon as parcels are delivered to the final logistic center, typically a city distribution center (CDC). Given the short delivery times requested by the customers, delivery operations typically need to start before all parcels expected to arrive that day are available at the CDC. This raises a question: whether to wait for more or all parcels to be delivered at the CDC or to start the delivery as soon as there is any available parcel and vehicle.
In this paper, we focus on the sequential decision making problem related to when to deliver parcels and which parcels to deliver under the assumption that the time at which parcels become available at the CDC (called release date) is stochastic and dynamic. We introduce a new problem called the Orienteering Problem with Stochastic and Dynamic Release Dates (DOP-rd) where a single and uncapacitated vehicle is serving customer requests with no time window, and the service has to finish within a deadline. The objective is to maximize the number of requests served within the deadline. We model the problem as a Markov decision process and present two approximation approaches for its solutions: a Policy Funciton Approximation (PFA) and a Value Function Approximation (VFA) approach. Both are based on scenario generations representing realizations of RDs and make use of a batch approach to approximate the value of future information, and specifically to approximate the number of requests served in future routes. We perform computational tests on a set of benchmark instances. We compare the two approaches described above with myopic approaches mimicking common practice and upper bounds related to perfect information. The results show that both PFA and VFA largely outperform myopic approaches and provide reasonable gaps with respect to the solution with perfect information.
E-commerce markets are booming at remarkable rates. It is reported that the e-commerce revenue saw a 10% increase in Europe in 2020 due to the pandemic. At the same time, the expectations of customers in terms of service quality are also increasing. An important feature of last-mile distribution is that its operations start as soon as parcels are delivered to the final logistic center, typically a city distribution center (CDC). Given the short delivery times requested by the customers, delivery operations typically need to start before all parcels expected to arrive that day are available at the CDC. This raises a question: whether to wait for more or all parcels to be delivered at the CDC or to start the delivery as soon as there is any available parcel and vehicle.
In this paper, we focus on the sequential decision making problem related to when to deliver parcels and which parcels to deliver under the assumption that the time at which parcels become available at the CDC (called release date) is stochastic and dynamic. We introduce a new problem called the Orienteering Problem with Stochastic and Dynamic Release Dates (DOP-rd) where a single and uncapacitated vehicle is serving customer requests with no time window, and the service has to finish within a deadline. The objective is to maximize the number of requests served within the deadline. We model the problem as a Markov decision process and present two approximation approaches for its solutions: a Policy Funciton Approximation (PFA) and a Value Function Approximation (VFA) approach. Both are based on scenario generations representing realizations of RDs and make use of a batch approach to approximate the value of future information, and specifically to approximate the number of requests served in future routes. We perform computational tests on a set of benchmark instances. We compare the two approaches described above with myopic approaches mimicking common practice and upper bounds related to perfect information. The results show that both PFA and VFA largely outperform myopic approaches and provide reasonable gaps with respect to the solution with perfect information.